The Crucial Role of Machine Learning Data Labeling in Business Growth
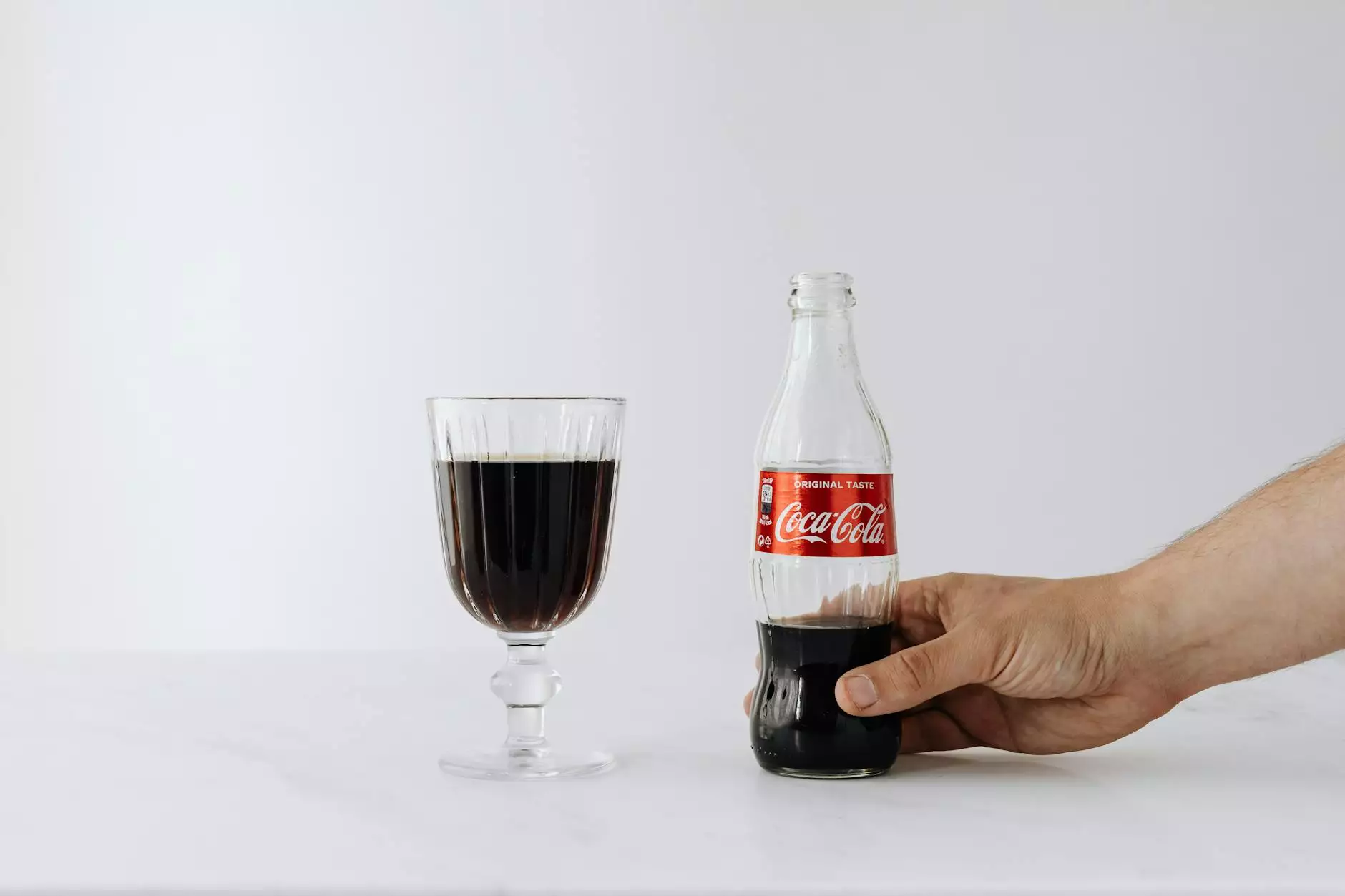
Machine learning data labeling is a pivotal aspect of modern business operations, particularly in sectors like home services and locksmithing. As businesses increasingly rely on data-driven decisions, understanding how to effectively label data for machine learning is essential for gaining a competitive edge.
What is Machine Learning Data Labeling?
At its core, machine learning data labeling refers to the process of classifying and annotating data to provide clear context for algorithms. This step is crucial for training machine learning models, which learn from examples to make predictions or decisions based on new data.
The Importance of Data Labeling
Data is the lifeblood of machine learning. However, raw data lacks the structure necessary for algorithms to interpret it effectively. Here’s why labeling is vital:
- Enhanced Model Accuracy: Accurately labeled data improves the precision of machine learning models, ensuring that they perform well on actual datasets.
- Faster Training Times: Properly labeled datasets speed up the training process, enabling businesses to bring products and services to market more quickly.
- Informed Decision Making: Businesses can leverage labeled data to derive insights that inform strategic decisions, optimizing operations in areas like marketing, pricing, and customer service.
How Machine Learning Data Labeling Works
The data labeling process typically involves several stages:
- Data Collection: Gathering data from various sources such as customer interactions, surveys, or social media.
- Data Annotation: Adding labels to the data, which could involve categorizing images, tagging text, or marking specific audio segments.
- Quality Assurance: Conducting reviews and audits to ensure the labels are accurate and reliable.
- Model Training: Using the labeled data to train machine learning models, which can then make predictions based on new, unlabeled data.
Types of Data Labeling Techniques
There are various techniques employed in machine learning data labeling, tailored to different types of data:
1. Classification
This involves categorizing data points into predefined classes. For example, in the locksmith industry, images of different keys can be labeled as "standard," "high-security," or "smart keys."
2. Object Detection
Used primarily in image processing, object detection labels specific elements within images. A locksmith might use this to identify security features in door locks.
3. Semantic Segmentation
This technique involves partitioning an image into parts and labeling each segment for more granular analysis, particularly useful for complex images.
Benefits of Machine Learning Data Labeling for Businesses
Implementing effective data labeling strategies can yield significant benefits for businesses in the home services and locksmith sectors:
1. Improved Customer Experience
With accurate data insights, businesses can better understand customer needs and preferences, leading to tailored services that enhance satisfaction.
2. Increased Operational Efficiency
Data labeling streamlines processes by automating routine tasks, enabling staff to focus on more complex issues that require human intervention.
3. Enhanced Marketing Strategies
By analyzing labeled customer data, businesses can develop targeted marketing campaigns that resonate more with their audience, thus improving conversion rates.
4. Competitive Advantage
Companies that leverage machine learning through labeled data are better positioned to adapt to market trends and consumer behaviors.
Best Practices for Effective Data Labeling
To harness the full potential of machine learning data labeling, businesses should adhere to several best practices:
- Define Clear Objectives: Before starting the labeling process, establish clear goals that align with business strategies.
- Utilize Quality Annotators: Hire trained professionals or leverage platforms with skilled annotators to enhance data labeling accuracy.
- Implement Feedback Loops: Continuously monitor and refine the labeling process based on model performance and feedback.
- Prioritize Data Quality: Ensure that the data collected for labeling is relevant, sufficient, and representative of the problem space.
Challenges in Machine Learning Data Labeling
While essential for machine learning success, data labeling comes with its own set of challenges:
1. Time-Consuming Process
The manual labeling of large datasets can be incredibly time-consuming, often leading to delays in project timelines.
2. Human Error
Even trained professionals can make errors in labeling, which can lead to inaccuracies in the model training process.
3. Resource Intensive
Data labeling requires significant resources in terms of both time and finances, particularly for small businesses.
The Future of Machine Learning Data Labeling
As technology evolves, so too does the field of machine learning data labeling. Here are some trends to watch:
1. Automation and AI Tools
With the rise of automated labeling tools, businesses can expect increased efficiency and reduced costs in data preparation processes.
2. Crowdsourcing Platforms
More companies are turning to crowdsourcing solutions for data labeling, allowing them to access a larger pool of annotators and expedite the process.
3. Emphasis on Bias Mitigation
As AI ethics becomes more critical, businesses will need to focus on mitigating bias in labeled datasets to ensure fairness in their systems.
Conclusion: Embracing the Importance of Machine Learning Data Labeling
In conclusion, machine learning data labeling is not just a technical task but a strategic initiative that can profoundly impact the success of businesses, particularly in the home services and locksmith sectors. By embracing effective labeling practices, organizations can enhance their operational capabilities, improve customer satisfaction, and ultimately drive growth and competitiveness in this data-driven age.
As the landscape of machine learning continues to evolve, businesses that recognize the value of accurate data labeling will be better equipped to meet the challenges of tomorrow and achieve sustained success.